Engineering Optimization: Theory and Practice, 5ed (An Indian Adaptation)
ISBN: 9789357461238
840 pages
For more information write to us at: acadmktg@wiley.com
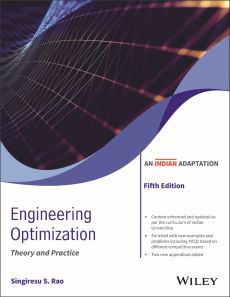
Description
This Indian adaptation of Engineering Optimization: Theory and Practice, offers new and updated material relevant to the Indian context. Two new appendices on Simulation and Network Analysis and Methods have been included. In line with the ethos of the original work, the text continues to provide a significant number of examples and problems that help illustrate the importance of the results presented in the text. More than a third of these examples and problems have been newly added or revised.
Preface to the Adapted Edition
Preface
Acknowledgment
About the Author
1 Introduction to Optimization
1.1 Introduction
1.2 Historical Development
1.3 Optimization in Engineering Practices
1.4 Defining an Optimization Problem
1.5 Classification of Optimization Problems
1.6 Tools and Techniques for Optimization
1.7 Engineering Optimization Literature
1.8 Challenges in Solving Optimization Problems
1.9 Solutions Using MATLAB
2 Classical Optimization Techniques
2.1 Introduction
2.2 Single-Variable Optimization
2.3 Multivariable Optimization with No Constraints
2.4 Multivariable Optimization with Equality Constraints
2.5 Multivariable Optimization with Inequality Constraints
2.6 Convex Programming Problem
3 Linear Programming I: Graphical and Simplex Method
3.1 Introduction
3.2 Applications of Linear Programming
3.3 Standard Form of a Linear Programming Problem
3.4 Geometry of Linear Programming Problems
3.5 Definitions and Theorems
3.6 Solution of a System of Linear Simultaneous Equations
3.7 Pivotal Reduction of a General System of Equations
3.8 Motivation of the Simplex Method
3.9 Simplex Algorithm
3.10 Two Phases of the Simplex Method
3.11 Big M Method
3.12 Solutions Using MATLAB
4 Linear Programming II: Additional Topics and Extensions
4.1 Introduction
4.2 Revised Simplex Method
4.3 Duality in Linear Programming
4.4 Decomposition Principle
4.5 Sensitivity or Postoptimality Analysis
4.6 Transportation Problem
4.7 Karmarkar’s Interior Method
4.8 Quadratic Programming
4.9 Solutions Using MATLAB
5 Nonlinear Programming I: One-Dimensional Minimization Methods
5.1 Introduction
5.2 Unimodal Function
5.3 Unrestricted Search
5.4 Exhaustive Search
5.5 Dichotomous Search
5.6 Interval Halving Method
5.7 Fibonacci Method
5.8 Golden Section Method
5.9 Comparison of Elimination Methods
5.10 Quadratic Interpolation Method
5.11 Cubic Interpolation Method
5.12 Direct Root Methods
5.13 Practical Considerations
5.14 Solutions Using MATLAB
6 Nonlinear Programming II: Unconstrained Optimization Techniques
6.1 Introduction
6.2 Random Search Methods
6.3 Grid Search Method
6.4 Univariate Method
6.5 Pattern Directions
6.6 Hooke and Jeeves’ Method
6.7 Powell’s Method
6.8 Simplex Method
6.9 Gradient of a Function
6.10 Steepest Descent (Cauchy) Method
6.11 Conjugate Gradient (Fletcher–Reeves) Method
6.12 Newton’s Method
6.13 Marquardt Method
6.14 Quasi-Newton Methods
6.15 Davidon–Fletcher–Powell Method
6.16 Broyden–Fletcher–Goldfarb–Shanno Method
6.17 Test Functions
6.18 Solutions Using MATLAB
7 Nonlinear Programming III: Constrained Optimization Techniques
7.1 Introduction
7.2 Characteristics of a Constrained Problem
7.3 Random Search Methods
7.4 Complex Method
7.5 Sequential Linear Programming
7.6 Basic Approach in the Methods of Feasible Directions
7.7 Zoutendijk’s Method of Feasible Directions
7.8 Rosen’s Gradient Projection Method
7.9 Generalized Reduced Gradient Method
7.10 Sequential Quadratic Programming
7.11 Transformation Techniques
7.12 Basic Approach of the Penalty Function Method
7.13 Interior Penalty Function Method
7.14 Convex Programming Problem
7.15 Exterior Penalty Function Method
7.16 Extrapolation Techniques in the Interior Penalty Function Method
7.17 Extended Interior Penalty Function Methods
7.18 Penalty Function Method for Problems with Mixed Equality and Inequality Constraints
7.19 Penalty Function Method for Parametric Constraints
7.20 Augmented Lagrange Multiplier Method
7.21 Checking the Convergence of Constrained Optimization Problems
7.22 Test Problems
7.23 Solutions Using MATLAB
8 Geometric Programming
8.1 Introduction
8.2 Posynomial
8.3 Unconstrained Minimization Problem
8.4 Solution of an Unconstrained Geometric Programming Program using Differential Calculus
8.5 Solution of an Unconstrained Geometric Programming Problem Using Arithmetic–Geometric Inequality
8.6 Primal–dual Relationship and Sufficiency Conditions in the Unconstrained Case
8.7 Constrained Minimization
8.8 Solution of a Constrained Geometric Programming Problem
8.9 Primal and Dual Programs in the Case of Less-than Inequalities
8.10 Geometric Programming with Mixed Inequality Constraints
8.11 Applications of Geometric Programming
9 Dynamic Programming
9.1 Introduction
9.2 Multistage Decision Processes
9.3 Concept of Suboptimization and Principle of Optimality
9.4 Computational Procedure in Dynamic Programming
9.5 Example Illustrating the Calculus Method of Solution
9.6 Example Illustrating the Tabular Method of Solution
9.7 Conversion of a Final Value Problem into an Initial Value Problem
9.8 Linear Programming as a Case of Dynamic Programming
9.9 Continuous Dynamic Programming
9.10 Engineering Applications
10 Integer Programming
10.1 Introduction
10.2 Graphical Representation
10.3 Gomory’s Cutting Plane Method
10.4 Balas’ Algorithm for Zero–One Programming Problems
10.5 Integer Polynomial Programming
10.6 Branch-and-Bound Method
10.7 Sequential Linear Discrete Programming
10.8 Generalized Penalty Function Method
11 Stochastic Programming
11.1 Introduction
11.2 Basic Concepts of Probability Theory
11.3 Formulating Stochastic Optimization Problems
11.4 Stochastic Linear Programming
11.5 Stochastic Nonlinear Programming
11.6 Stochastic Geometric Programming
11.7 Applications and Examples of Stochastic Programming
12 Optimal Control and Optimality Criteria Methods
12.1 Introduction
12.2 Calculus of Variations
12.3 Optimal Control Theory
12.4 Optimality Criteria Methods
13 Methods of Optimization using Algorithm
13.1 Introduction
13.2 Genetic Algorithms
13.3 Simulated Annealing
13.4 Particle Swarm Optimization
13.5 Ant Colony Optimization
13.6 Optimization of Fuzzy Systems
13.7 Neural-Network-Based Optimization
14 Metaheuristic Optimization Methods
14.1 Definitions
14.2 Metaphors Associated with Metaheuristic Optimization Methods
14.3 Details of Representative Metaheuristic Algorithms
15 Practical Aspects of Optimization
15.1 Introduction
15.2 Reduction of Size of an Optimization Problem
15.3 Fast Reanalysis Techniques
15.4 Derivatives of Static Displacements and Stresses
15.5 Derivatives of Eigenvalues and Eigenvectors
15.6 Derivatives of Transient Response
15.7 Sensitivity of Optimum Solution to Problem Parameters
16 Multilevel and Multiobjective Optimization
16.1 Introduction
16.2 Multilevel Optimization
16.3 Parallel Processing
16.4 Multi Objective Optimization
16.5 Solutions Using MATLAB
17 Solution of Optimization Problems Using MATLAB
17.1 Introduction
17.2 Solution of General Nonlinear Programming Problems
17.3 Solution of Linear Programming Problems
17.4 Solution of LP Problems Using Interior Point Method
17.5 Solution of Quadratic Programming Problems
17.6 Solution of One-Dimensional Minimization Problems
17.7 Solution of Unconstrained Optimization Problems
17.8 Solution of Constrained Optimization Problems
17.9 Solution of Binary Programming Problems
17.10 Solution of Multiobjective Problems
References and Bibliography
Problems
A Convex and Concave Functions
B Some Computational Aspects of Optimization
B.1 Choice of Method
B.2 Comparison of Unconstrained Methods
B.3 Comparison of Constrained Methods
B.4 Availability of Computer Programs
B.5 Scaling of Design Variables and Constraints
B.6 Computer Programs for Modern Methods of Optimization
C Introduction to MATLAB®
C.1 Features and Special Characters
C.2 Defining Matrices in MATLAB
C.3 Creating m-Files
C.4 Optimization Toolbox
D Simulation
D.1 Definition
D.2 Types of Simulation
D.3 Steps in the Simulation Process
D.4 Advantages and Disadvantages of Simulation
D.5 Stochastic Simulation and Random Numbers
D.6 Simulation of Inventory Problems
D.7 Simulation of Queueing Problems
D.8 Simulation of Maintenance Problems
D.9 Applications of Simulation
E Network Analysis and Methods
E.1 Introduction
E.2 Minimum Spanning Tree
E.3 Shortest Path Problem