Fundamentals of Data Science Using Python and R
ISBN: 9789363860759
276 pages
For more information write to us at: acadmktg@wiley.com
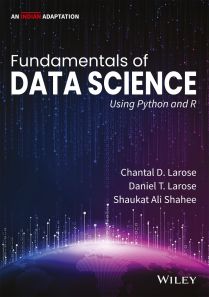
Description
Fundamentals of Data Science Using Python and R is an essential resource for students and professionals eager to explore data science with Python and R, two of the most popular open-source tools. The book covers the entire Data Science Methodology—from problem understanding to model deployment—and has been widely praised for its clarity and practicality. This adapted edition retains the core structure of the original, while enhancing end-of-chapter questions to suit the Indian academic environment. New examples and exercises focus on India-specific datasets, encouraging students to apply their knowledge to real-world scenarios relevant to India’s socio-economic and technological contexts. This hands-on approach ensures students gain both theoretical understanding and practical skills for a data-driven world.
PREFACE TO THE ADAPTED EDITION
PREFACE TO THE US EDITION
ACKNOWLEDGMENTS
ABOUT THE AUTHORS
CHAPTER 1 INTRODUCTION TO DATA SCIENCE
1.1 Why Data Science?
1.2 What Is Data Science?
1.3 The Data Science Methodology
1.4 Data Science Tasks
1.4.1 Description
1.4.2 Estimation
1.4.3 Classification
1.4.4 Clustering
1.4.5 Prediction
1.4.6 Association
Exercises
CHAPTER 2 THE BASICS OF PYTHON AND R
2.1 Downloading Python
2.2 Basics of Coding in Python
2.2.1 Using Comments in Python
2.2.2 Executing Commands in Python
2.2.3 Importing Packages in Python
2.2.4 Getting Data into Python
2.2.5 Saving Output in Python
2.2.6 Accessing Records and Variables in Python
2.2.7 Setting Up Graphics in Python
2.3 Downloading R and Rstudio
2.4 Basics of Coding in R
2.4.1 Using Comments in R
2.4.2 Executing Commands in R
2.4.3 Importing Packages in R
2.4.4 Getting Data into R
2.4.5 Saving Output in R
2.4.6 Accessing Records and Variables in R
References
Exercises
CHAPTER 3 DATA PREPARATION
3.1 The Bank Marketing Data Set
3.2 The Problem Understanding Phase
3.2.1 Clearly Enunciate the Project Objectives
3.2.2 Translate These Objectives into a Data Science Problem
3.3 Data Preparation Phase
3.4 Adding an Index Field
3.4.1 How to Add an Index Field Using Python
3.4.2 How to Add an Index Field Using R
3.5 Changing Misleading Field Values
3.5.1 How to Change Misleading Field Values Using Python
3.5.2 How to Change Misleading Field Values Using R
3.6 Reexpression of Categorical Data as Numeric
3.6.1 How to Reexpress Categorical Field Values Using Python
3.6.2 How to Reexpress Categorical Field Values Using R
3.7 Standardizing the Numeric Fields
3.7.1 How to Standardize Numeric Fields Using Python
3.7.2 How to Standardize Numeric Fields Using R
3.8 Identifying Outliers
3.8.1 How to Identify Outliers Using Python
3.8.2 How to Identify Outliers Using R
References
Exercises 45
CHAPTER 4 EXPLORATOR Y DATA ANALYSIS
4.1 Eda Versus HT
4.2 Bar Graphs with Response Overlay
4.2.1 How to Construct a Bar Graph with Overlay Using Python
4.2.2 How to Construct a Bar Graph with Overlay Using R
4.3 Contingency Tables
4.3.1 How to Construct Contingency Tables Using Python
4.3.2 How to Construct Contingency Tables Using R
4.4 Histograms with Response Overlay
4.4.1 How to Construct Histograms with Overlay Using Python
4.4.2 How to Construct Histograms with Overlay Using R
4.5 Binning Based on Predictive Value
4.5.1 How to Perform Binning Based on Predictive Value Using Python
4.5.2 How to Perform Binning Based on Predictive Value Using R
References
Exercises
CHAPTER 5 PREPARING TO MODEL THE DATA
5.1 The Story So Far
5.2 Partitioning the Data
5.2.1 How to Partition the Data in Python
5.2.2 How to Partition the Data in R
5.3 Validating Your Partition
5.4 Balancing the Training Data Set
5.4.1 How to Balance the Training Data Set in Python
5.4.2 How to Balance the Training Data Set in R
5.5 Establishing Baseline Model Performance
References
Exercises
CHAPTER 6 DECISION TREES
6.1 Introduction to Decision Trees
6.2 Classification and Regression Trees
6.2.1 How to Build CART Decision Trees Using Python
6.2.2 How to Build CART Decision Trees Using R
6.3 The C5.0 Algorithm for Building Decision Trees
6.3.1 How to Build C5.0 Decision Trees Using Python
6.3.2 How to Build C5.0 Decision Trees Using R
6.4 Random Forests
6.4.1 How to Build Random Forests in Python
6.4.2 How to Build Random Forests in R
References
Exercises
CHAPTER 7 MODEL EVALUATION
7.1 Introduction to Model Evaluation
7.2 Classification Evaluation Measures
7.3 Sensitivity and Specificity
7.4 Precision, Recall, and Fβ Scores
7.5 Method for Model Evaluation
7.6 An Application of Model Evaluation
7.6.1 How to Perform Model Evaluation Using R
7.7 Accounting for Unequal Error Costs
7.7.1 Accounting for Unequal Error Costs Using R
7.8 Comparing Models with and Without Unequal Error Costs
7.9 Data-Driven Error Costs
Exercises
CHAPTER 8 NAÏVE BAYES CLASSIFICATION
8.1 Introduction to Naïve Bayes
8.2 Bayes Theorem
8.3 Maximum a Posteriori Hypothesis
8.4 Class Conditional Independence
8.5 Application of Naïve Bayes Classification
8.5.1 Naïve Bayes in Python
8.5.2 Naïve Bayes in R
References
Exercises
CHAPTER 9 NEURAL NETWORKS
9.1 Introduction to Neural Networks
9.2 The Neural Network Structure
9.3 Connection Weights and the Combination Function
9.4 The Sigmoid Activation Function
9.5 Backpropagation
9.6 An Application of a Neural Network Model
9.7 Interpreting the Weights in a Neural Network Model
9.8 How to Use Neural Networks in R
9.9 How to Use Neural Networks in Python
References
Exercises
CHAPTER 10 CLUSTERING
10.1 What Is Clustering?
10.2 Introduction to the k-Means Clustering Algorithm
10.3 An Application of k-Means Clustering
10.4 Cluster Validation
10.5 How to Perform k-Means Clustering Using Python
10.5.1 k-Means Python Example Using Sklearn
10.6 How to Perform k-Means Clustering Using R
Exercises
CHAPTER 11 REGRESSION MODELING
11.1 The Estimation Task
11.2 Descriptive Regression Modeling
11.3 An Application of Multiple Regression Modeling
11.4 How to Perform Multiple Regression Modeling Using Python
11.5 How to Perform Multiple Regression Modeling Using Sklearn Python
11.6 How to Perform Multiple Regression Modeling Using R
11.7 Model Evaluation for Estimation
11.7.1 How to Perform Stepwise Regression Using Python
11.7.2 How to Perform Estimation Model Evaluation Using Python
11.7.3 How to Perform Estimation Model Evaluation Using R
11.8 Stepwise Regression
11.8.1 How to Perform Stepwise Regression Using R
11.9 Baseline Models for Regression
References
Exercises
CHAPTER 12 DIMENSION REDUCTION
12.1 The Need for Dimension Reduction
12.2 Multicollinearity
12.3 Identifying Multicollinearity Using Variance Inflation Factors
12.3.1 How to Identify Multicollinearity Using Python
12.3.2 How to Identify Multicollinearity in R
12.4 Principal Components Analysis
12.5 An Application of Principal Components Analysis
12.6 How Many Components Should We Extract?
12.6.1 The Eigenvalue Criterion
12.6.2 The Proportion of Variance Explained Criterion
12.7 Performing PCA with k = 4
12.8 Validation of the Principal Components
12.9 How to Perform Principal Components Analysis Using Python
12.10 How to Perform Principal Components Analysis Using R
12.11 When Is Multicollinearity Not a Problem?
References
Exercises
CHAPTER 13 GENERALIZED LINEAR MODELS
13.1 An Overview of General Linear Models
13.2 Linear Regression As a General Linear Model
13.3 Logistic Regression As a General Linear Model
13.4 An Application of Logistic Regression Modeling
13.4.1 How to Perform Logistic Regression Using Python
13.4.2 How to Perform Logistic Regression Using R
13.5 Poisson Regression
13.6 An Application of Poisson Regression Modeling
13.6.1 How to Perform Poisson Regression Using Python
13.6.2 How to Perform Poisson Regression Using R
Reference
Exercises
CHAPTER 14 ASSOCIATION RULES
14.1 Introduction to Association Rules
14.2 A Simple Example of Association Rule Mining
14.3 Support, Confidence, and Lift
14.4 Mining Association Rules
14.4.1 How to Mine Association Rules Using R
14.5 Confirming Our Metrics
14.6 The Confidence Difference Criterion
14.6.1 How to Apply the Confidence Difference Criterion Using R
14.7 The Confidence Quotient Criterion
14.7.1 How to Apply the Confidence Quotient Criterion Using R
Valediction
References
Exercises
APPENDIX DATA SUMMARIZATION AND VISUALIZATION
Part 1 Summarization 1: Building Blocks of Data Analysis
Part 2 Visualization: Graphs and Tables for Summarizing and Organizing Data
A.1 Categorical Variables
A.2 Quantitative Variables
Part 3 Summarization 2: Measures of Center, Variability, and Position
Part 4 Summarization and Visualization of Bivariate Relationships
INDEX